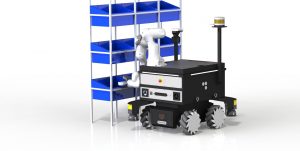
Work is progressing towards the realisation of the initial hardware platform to be used in DARKO. The fleet of three robots is under construction, and will look similar to this concept drawing. Another notable exciting update is that we have developed a new prototype of a variable-stiffness wrist, which will improve efficiency and performance for both grasping and throwing.
We have recently contributed two benchmarks that will make it easier to fairly and comprehensively compare new state-of-the-art methods for motion prediction and motion planning. For robots to work well (both safely and efficiently) together with people, it is essential that they can quickly generate safe and smooth paths for going from A to B. While driving, they also need to be able to see the people around them and anticipate where they are going next. Our new benchmarking tools, dubbed Atlas and Bench-MR, were recently published at two scientific conferences.
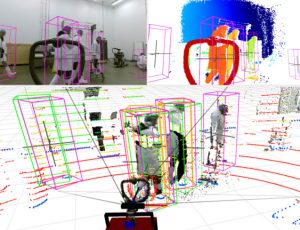
We also recently published new work that helps to answer the question “which sensor-algorithm combination is best suited for a person detection task at hand?” Advances in sensing and learning algorithms have led to increasingly mature solutions for human detection by robots. Despite this progress, this simple question remains hard to answer. We have conducted a systematic analysis of sensor-algorithm combinations typically used in robotics, and our results point to relevant open challenges for further research and provide valuable support to practitioners for the design of their robot system.
Finally, we have published novel work to detect when an object risks sliding out of the grasp of a robotic hand, by combining tactile sensing and machine learning. If the robot can predict that the grip is slipping, it can also re-grasp to get a better grip. This type of functionality will be very important for the DARKO use-case, making it easier to delicately handle, and throw, objects.