Giuseppe Averta, Federica Barontini, Irene Valdambrini, Paolo Cheli, Davide Bacciu, Matteo Bianchi
Learning to prevent grasp failure with soft hands: from on-lineprediction to dual-arm grasp recovery
Advanced Intelligent Systems
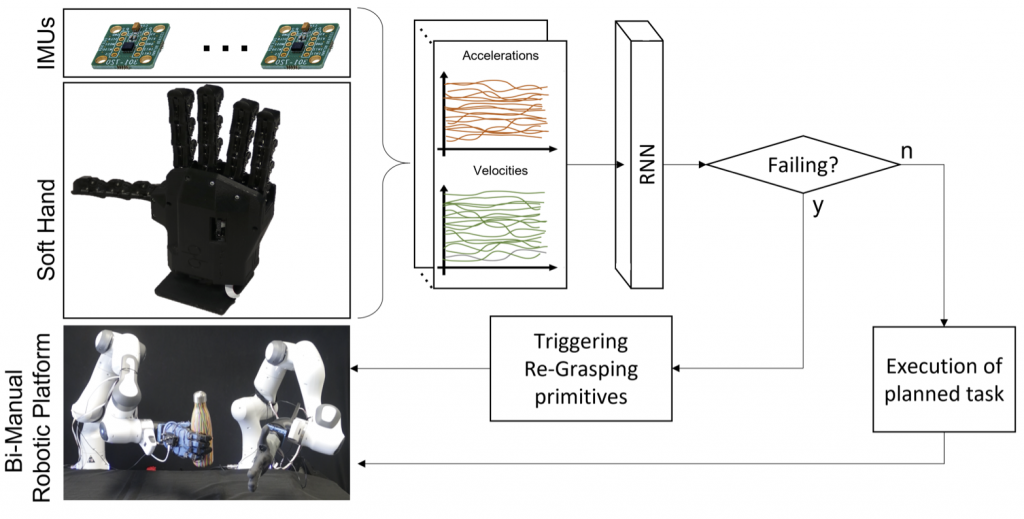
Abstract
Soft-hands allow to simplify the grasp planning to achieve a successful grasp, thanks to their intrinsic adaptability. At the same time, their usage poses new challenges, related to the adoption of classical sensing techniques originally developed for rigid end-defectors, which provide fundamental information, e.g. to detect object slippage. Under this regard, model-based approaches for the processing of the gathered information are hard to use, due to the difficulties in modelling hand-object interaction when softness is involved. To overcome these limitations, in this paper we propose to combine distributed tactile sensing and machine learning (Recurrent Neural Network – RNN) to detect sliding conditions for a soft robotic hand mounted on a robotic manipulator, targeting the prediction of the grasp failure event and the direction of sliding. The outcomes of these predictions allow for an-on line triggering of a compensatory action performed with a second robotic arm-hand system, to prevent the failure. Despite the fact that the network was trained only with spherical and cylindrical objects, we demonstrate high generalization capabilities of our framework, achieving a correct prediction of the failure direction in 75% of cases, and a 85% of successful re-grasps, for a selection of twelve objects of common use.
@Article{averta2021failure, author = {Averta, Giuseppe, Barontini, Federica, Valdambrini, Irene, Cheli, Paolo, Bacciu, Davide, Bianchi, Matteo}, date = {2021}, journaltitle = {Wiley Advanced Intelligent Systems}, title = {Learning to prevent grasp failure with soft hands: from on-lineprediction to dual-arm grasp recovery}, }