Tiago Rodrigues de Almeida, Andrey Rudenko, Tim Schreiter, Yufei Zhu, Eduardo Gutierrez Maestro, Lucas Morillo-Mendez, Tomasz P Kucner, Oscar Martinez Mozos, Martin Magnusson, Luigi Palmieri, Kai O Arras, and Achim J Lilienthal
Proceedings of the IEEE/CVF International Conference on Computer Vision
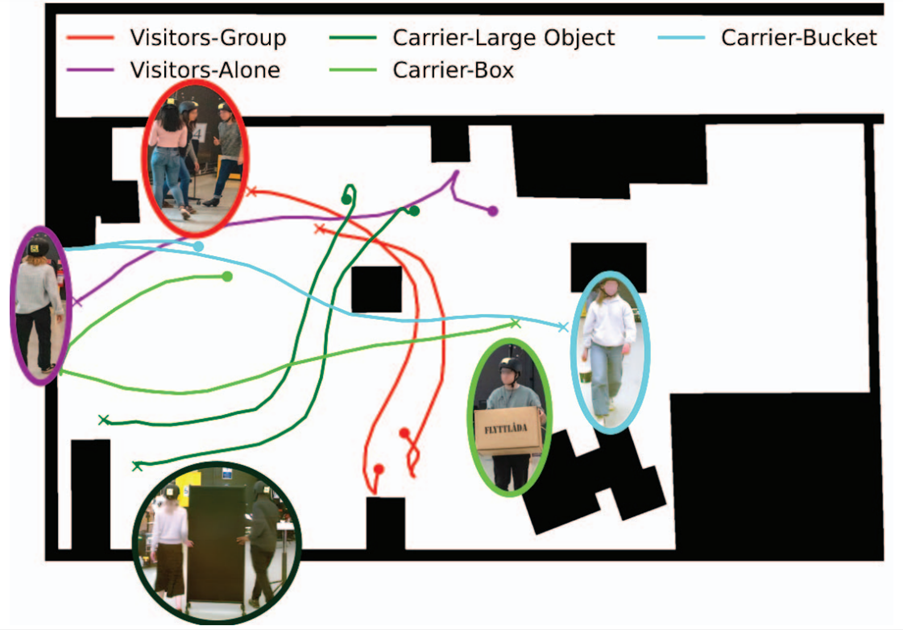
Abstract
Autonomous systems, that need to operate in human environments and interact with the users, rely on understanding and anticipating human activity and motion. Among the many factors which influence human motion, semantic attributes, such as the roles and ongoing activities of the detected people, provide a powerful cue on their future motion, actions, and intentions. In this work we adapt several popular deep learning models for trajectory prediction with labels corresponding to the roles of the people. To this end we use the novel THOR-Magni dataset, which captures human activity in industrial settings and includes the relevant semantic labels for people who navigate complex environments, interact with objects and robots, work alone and in groups. In qualitative and quantitative experiments we show that the role-conditioned LSTM, Transformer, GAN and VAE methods can effectively incorporate the semantic categories, better capture the underlying input distribution and therefore produce more accurate motion predictions in terms of Top-K ADE/FDE and log-likelihood metrics.
@inproceedings{de2023thor, title={THOR-Magni: Comparative Analysis of Deep Learning Models for Role-Conditioned Human Motion Prediction}, author={de Almeida, Tiago Rodrigues and Rudenko, Andrey and Schreiter, Tim and Zhu, Yufei and Maestro, Eduardo Gutierrez and Morillo-Mendez, Lucas and Kucner, Tomasz P and Mozos, Oscar Martinez and Magnusson, Martin and Palmieri, Luigi and others}, booktitle={Proceedings of the IEEE/CVF International Conference on Computer Vision}, pages={2200--2209}, year={2023} }