Alessandro Palleschi, Franco Angelini, Chiara Gabellieri, Do Won Park, Lucia Pallottino, Antonio Bicchi, and Manolo Garabini
IEEE Transactions on Robotics
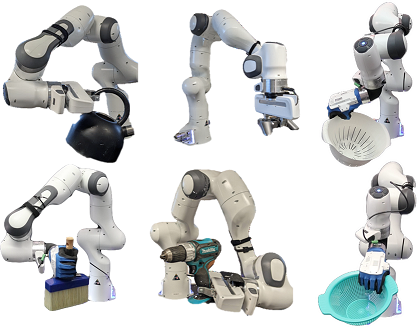
Abstract
With the improvements in their computational and physical intelligence, robots are now capable of operating in real-world environments. However, manipulation and grasping capabilities are still areas that require significant improvements. To address this, we introduce a new data-driven grasp planning algorithm called Grasp it Like a Pro 2.0. This algorithm utilizes a small number of human demonstrations to teach a robot how to grasp arbitrary objects. By decomposing objects into basic shapes, our algorithm generates candidate grasps that can generalize to different object’s geometry. The algorithm selects the grasp to execute based on a selection policy that maximizes a novel grasp quality metric introduced in this article. This metric considers the complex interdependencies between the predicted grasp, the local approximation produced by the basic shape decomposition, and the gripper used. We evaluate our approach against multiple baselines using different grippers and objects. The results demonstrate the effectiveness of our method in generating and selecting high-quality and reliable grasps. With a soft underactuated robotic hand, our algorithm achieves a 94.0% success rate in 150 grasps across 30 different objects. Similarly, with a rigid gripper, it achieves an 85.0% success rate in 80 grasps across 16 different objects.
@article{palleschi2023grasp, author={Palleschi, Alessandro and Angelini, Franco and Gabellieri, Chiara and Park, Do Won and Pallottino, Lucia and Bicchi, Antonio and Garabini, Manolo}, journal={IEEE Transactions on Robotics}, title={Grasp It Like a Pro 2.0: A Data-Driven Approach Exploiting Basic Shape Decomposition and Human Data for Grasping Unknown Objects}, year={2023}, volume={39}, number={5}, pages={4016-4036}, doi={10.1109/TRO.2023.3286115}}