Yufei Zhu, Andrey Rudenko, Tomasz P. Kucner, Achim J. Lilienthal, and Martin Magnusson
Proceedings of the 5th LHMP Workshop held in conjunction with 40th IEEE International Conference on Robotics and Automation (ICRA)
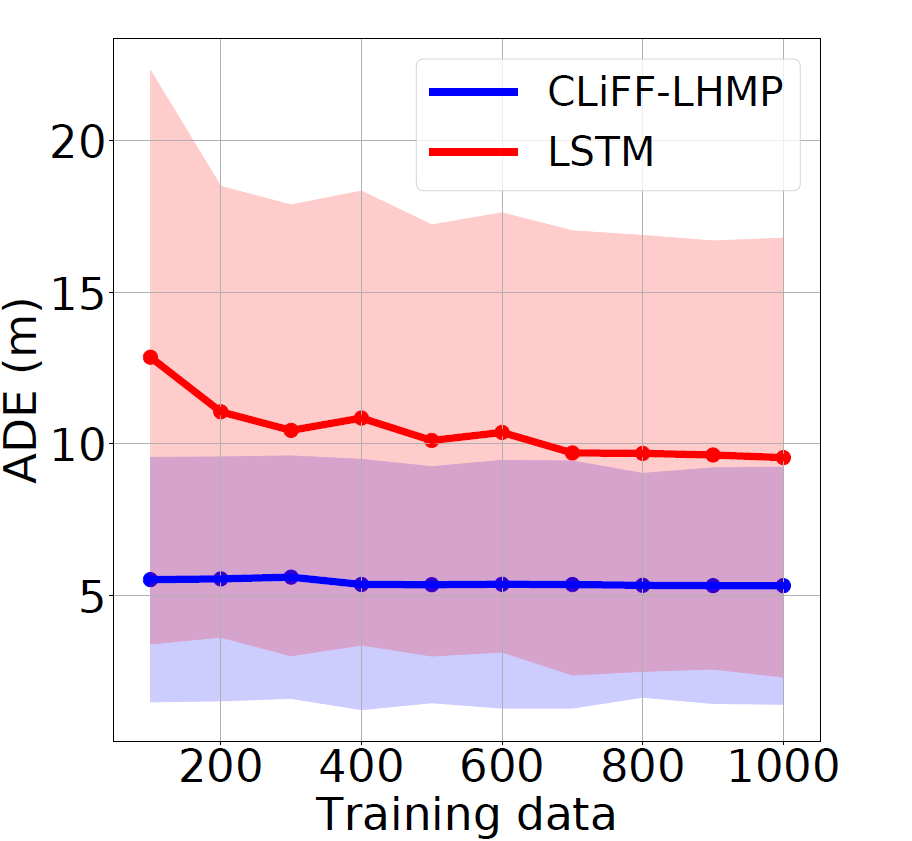
Abstract
Human motion prediction is essential for the safe and smooth operation of mobile service robots and intelligent vehicles around people. Commonly used neural network-based approaches often require large amounts of complete trajectories to represent motion dynamics in complex semantically rich spaces. This requirement may complicate deployment of physical systems in new environments, especially when the data is being collected online from onboard sensors. In this paper we explore a data-efficient alternative using maps of dynamics (MoD) to represent place-dependent multi-modal spatial motion patterns, learned from prior observations. Our approach can perform efficient human motion prediction in the long-term perspective of up to 60 seconds. We quantitatively evaluate its accuracy with limited amount of training data in comparison to an LSTM-based baseline, and qualitatively show that the predicted trajectories reflect the natural semantic properties of the environment, e.g. the locations of short- and long-term goals, navigation in narrow passages, around obstacles, etc.
@inproceedings{zhu2023data, title={A Data-Efficient Approach for Long-Term Human Motion Prediction Using Maps of Dynamics}, author={Zhu, Yufei and Rudenko, Andrey and Kucner, Tomasz P and Lilienthal, Achim J and Magnusson, Martin}, booktitle={IEEE ICRA 2023 Workshop Proceedings: 5th Workshop on Long-term Human Motion Prediction (LHMP 2023)}, year={2023} }