Tiago Rodrigues de Almeida, Yufei Zhu, Andrey Rudenko, Tomasz P. Kucner, Johannes A. Stork, Martin Magnusson and Achim J. Lilienthal
IEEE Robotics and Automation Letters
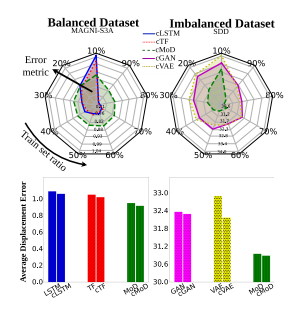
Abstract
Robots and other intelligent systems navigating in complex dynamic environments should predict future actions and
intentions of surrounding agents to reach their goals efficiently and avoid collisions. The dynamics of those agents strongly depends on their tasks, roles, or observable labels. Class-conditioned motion prediction is thus an appealing way to reduce forecast uncertainty and get more accurate predictions for heterogeneous agents. However, this is hardly explored in the prior art, especially for mobile robots and in limited data applications. In this paper, we analyse different class-conditioned trajectory prediction methods on two datasets.We propose a set of conditional pattern-based and efficient deep learning-based baselines, and evaluate their performance on robotics and outdoors datasets (THÖR-MAGNI and Stanford Drone Dataset). Our experiments show that all methods improve accuracy in most of the settings when considering class labels. More importantly, we observe that there are significant differences when learning from imbalanced datasets, or in new environments where sufficient data is not available. In particular, we find that deep learning methods perform better on balanced datasets, but in applications with limited data, e.g., cold start of a robot in a new environment, or imbalanced classes, pattern-based methods may be preferable.
@ARTICLE{10545544, author={de Almeida, Tiago Rodrigues and Zhu, Yufei and Rudenko, Andrey and Kucner, Tomasz P. and Stork, Johannes A. and Magnusson, Martin and Lilienthal, Achim J.}, journal={IEEE Robotics and Automation Letters}, title={Trajectory Prediction for Heterogeneous Agents: A Performance Analysis on Small and Imbalanced Datasets}, year={2024}, volume={9}, number={7}, pages={6576-6583}, keywords={Trajectory;Deep learning;Robots;Roads;Navigation;Generative adversarial networks;Dynamics;Datasets for human motion;deep learning methods;human and humanoid motion analysis and synthesis;human detection and tracking}, doi={10.1109/LRA.2024.3408510}}