Sebastian Koch, Pedro Hermosilla, Narunas Vaskevicius, Mirco Colosi, and Timo Ropinski
Proceedings of IEEE Winter Conference on Applications of Computer Vision (WACV)
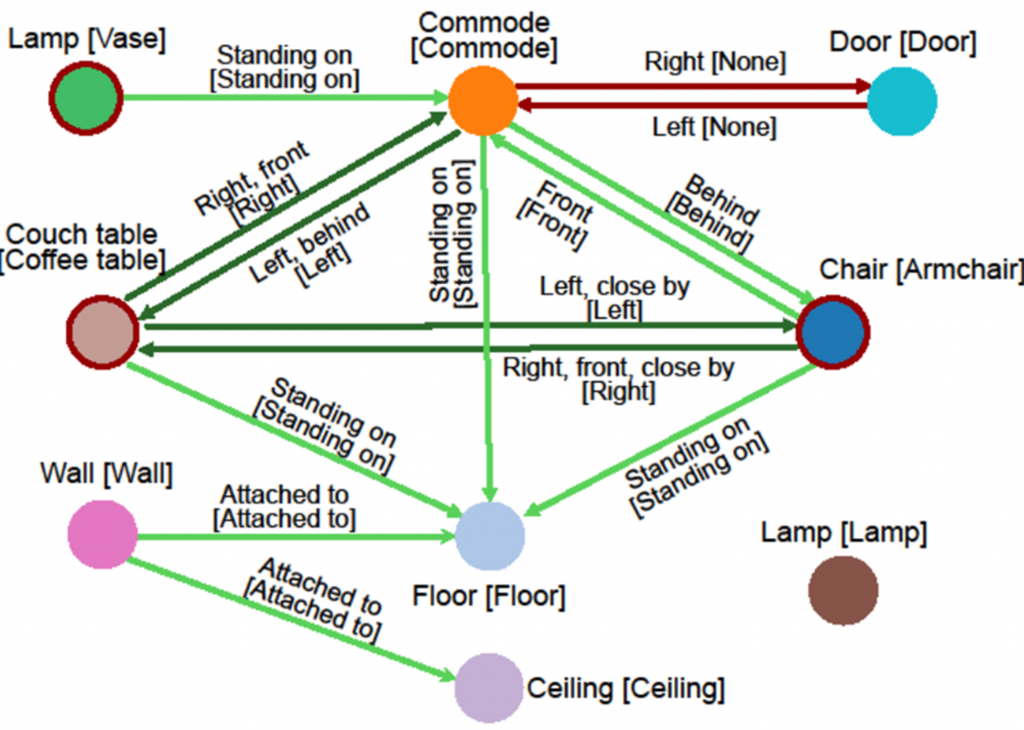
Abstract
In the field of 3D scene understanding, 3D scene graphs have emerged as a new scene representation that combines geometric and semantic information about objects and their relationships. However, learning semantic 3D scene graphs in a fully supervised manner is inherently difficult as it requires not only object-level annotations but also relationship labels. While pre-training approaches have helped to boost the performance of many methods in various fields, pre-training for 3D scene graph prediction has received little attention. Furthermore, we find in this paper that classical contrastive point cloud-based pre-training approaches are ineffective for 3D scene graph learning. To this end, we present SGRec3D, a novel self-supervised pre-training method for 3D scene graph prediction. We propose to reconstruct the 3D input scene from a graph bottleneck as a pretext task. Pre-training SGRec3D does not require object relationship labels, making it possible to exploit large-scale 3D scene understanding datasets, which were off-limits for 3D scene graph learning before. Our experiments demonstrate that in contrast to recent point cloud-based pre-training approaches, our proposed pre-training improves the 3D scene graph prediction considerably, which results in SOTA performance, outperforming other 3D scene graph models by +10% on object prediction and +4% on relationship prediction. Additionally, we show that only using a small subset of 10% labeled data during fine-tuning is sufficient to outperform the same model without pre-training.
@article{koch2023sgrec, title={SGRec3D: Self-Supervised 3D Scene Graph Learning via Object-Level Scene Reconstruction}, author={Koch, Sebastian and Hermosilla, Pedro and Vaskevicius, Narunas and Colosi, Mirco and Ropinski, Timo}, booktitle = {IEEE Winter Conference on Applications of Computer Vision (WACV)}, year={2024}, month={January} }