Yash Goel, Narunas Vaskevicius, Luigi Palmieri, Nived Chebrolu, Kai Oliver Arras, and Cyrill Stachniss
Proceedings of the IEEE/RSJ International Conference on Intelligent Robots and Systems (IROS)
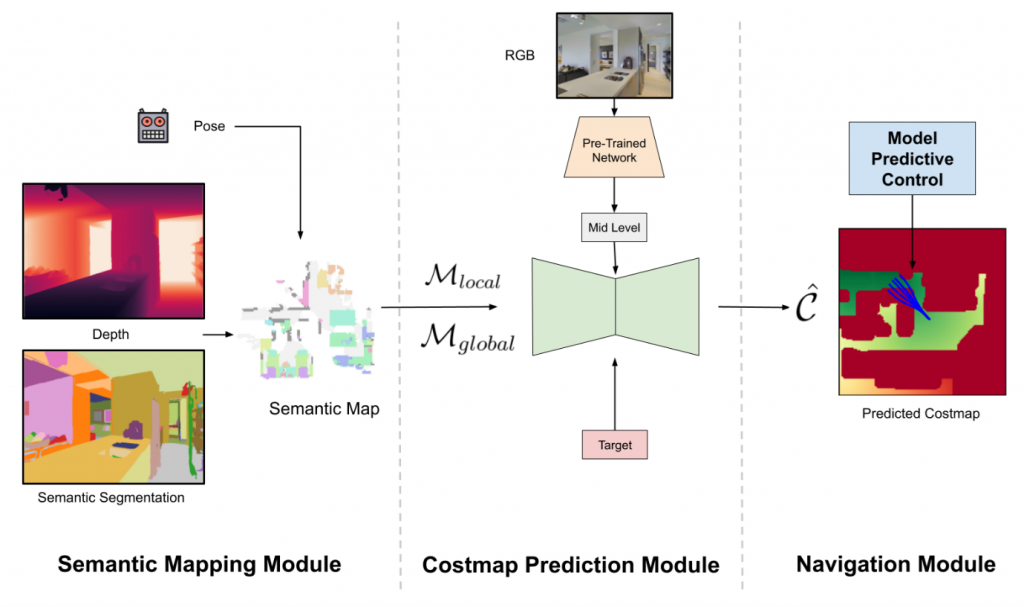
Abstract
We investigate the task of object goal navigation in unknown environments where a target object is given as a semantic label (e.g. find a couch). This task is challenging as it requires the robot to consider the semantic context in diverse settings (e.g. TVs are often nearby couches). Most of the prior work tackles this problem under the assumption of a discrete action policy whereas we present an approach with continuous control which brings it closer to real world applications. In this paper, we use information-theoretic model predictive control on dense cost maps to bring object goal navigation closer to real robots with kinodynamic constraints. We propose a deep neural network framework to learn cost maps that encode semantic context and guide the robot towards the target object. We also present a novel way of fusing mid-level visual representations in our architecture to provide additional semantic cues for cost map prediction. The experiments show that our method leads to more efficient and accurate goal navigation with higher quality paths than the reported baselines. The results also indicate the importance of mid-level representations for navigation by improving the success rate by 8 percentage points.
@inproceedings{goel2023iros, author = {Y. Goel and N. Vaskevicius and L. Palmieri and N. Chebrolu and K.O. Arras and C. Stachniss}, title = {{Semantically Informed MPC for Context-Aware Robot Exploration}}, booktitle = {IEEE/RSJ International Conference on Intelligent Robots and Systems (IROS)}, year = {2023}, }