Tiago Rodrigues de Almeida, Andrey Rudenko, Tim Schreiter, Yufei Zhu, Eduardo Gutierrez Maestro, Lukas Heuer, Luigi Palmieri, Andrey Rudenko, Anna Mannucci, Martin Magnusson, and Kai Oliver Arras
Proceedings of the IROS Conference
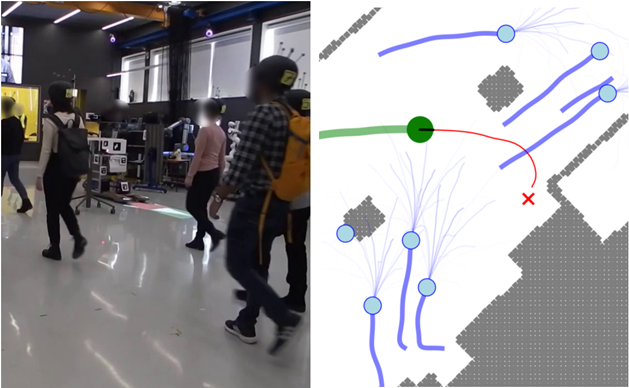
Abstract
For robots navigating in dynamic environments, exploiting and understanding uncertain human motion prediction is key to generate efficient, safe and legible actions. The robot may perform poorly and cause hindrances if it does not reason over possible, multi-modal future social interactions. With the goal of enhancing autonomous navigation in cluttered environments, we propose a novel formulation for nonlinear model predictive control including multi-modal predictions of human motion. As a result, our approach leads to less conservative, smooth and intuitive human-aware navigation with reduced risk of collisions, and shows a good balance between task efficiency, collision avoidance and human comfort. To show its effectiveness, we compare our approach against the state of the art in crowded simulated environments, and with real-world human motion data from the THÖR dataset. This comparison shows that we are able to improve task efficiency, keep a larger distance to humans and significantly reduce the collision time, when navigating in cluttered dynamic environments. Furthermore, the method is shown to work robustly with different state-of-the-art human motion predictors.
@inproceedings{heuer2023iros, author = {L. Heuer and L. Palmieri and A. Rudenko and A. Mannucci and M. Magnusson and K.O. Arras}, title = {{Proactive Model Predictive Control with Multi-Modal Human Motion Prediction in Cluttered Dynamic Environments}}, booktitle = {IEEE/RSJ International Conference on Intelligent Robots and Systems (IROS)}, year = {2023}, }