Shih-Min Yang, Martin Magnusson, Johannes Andreas Stork, and Todor Stoyanov
Proceedings of IROS 2023 Workshop on Leveraging Models for Contact-Rich Manipulation
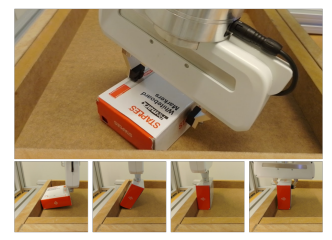
Abstract
In many real-world robotic grasping tasks, the target object is not directly graspable because all possible grasps are obstructed. In these cases, single-shot grasp planning will not work, and the object must first be manipulated into a configuration that allows for grasping. Our proposed method ED-PAP solves this problem by learning a sequence of actions that exploit constraints in the environment to change the object’s pose. Concretely, we employ hierarchical reinforcement learning to distill controllers that apply a sequence of learned parameterized feedback-based action primitives. By learning a policy that decides on a sequence of manipulation actions, we can generate a complex manipulation behavior that exploits physical interaction between the object, the gripper, and the environment. Designing such a complex behavior analytically would be difficult. Our hierarchical policy model operates directly on depth perception data without the need for object detection or pose estimation. We demonstrate and evaluate our approach for a clutter-free table-top scenario where we manipulate a box-shaped object and use interactions with the environment to re-orient the object in a graspable configuration.
@inproceedings{yang2023data, title={Data-driven Grasping and Pre-grasp Manipulation Using Hierarchical Reinforcement Learning with Parameterized Action Primitives}, author={Yang, Shih-Min and Magnusson, Martin and Stork, Johannes Andreas and Stoyanov, Todor}, booktitle={IROS 2023 Workshop on Leveraging Models for Contact-Rich Manipulation}, year={2023} }