Yufei Zhu, Andrey Rudenko, Tomasz P. Kucner, Luigi Palmieri, Kai O. Arras, Achim J. Lilienthal, and Martin Magnusson
Proceedings of IEEE/RSJ International Conference on Intelligent Robots and Systems (IROS)
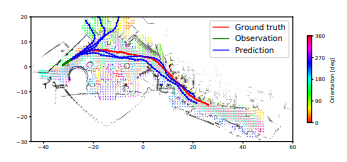
Abstract
Human motion prediction is important for mobile service robots and intelligent vehicles to operate safely and smoothly around people. The more accurate predictions are, particularly over extended periods of time, the better a system can, e.g., assess collision risks and plan ahead. In this paper, we propose to exploit maps of dynamics (MoDs, a class of general representations of place-dependent spatial motion patterns, learned from prior observations) for long-term human motion prediction (LHMP). We present a new MoD-informed human motion prediction approach, named CLiFF-LHMP, which is data effcient, explainable, and insensitive to errors from an upstream tracking system. Our approach uses CLiFF-map, a specifc MoD trained with human motion data recorded in the same environment. We bias a constant velocity prediction with samples from the CLiFF-map to generate multi-modal trajectory predictions. In two public datasets we show that this algorithm outperforms the state of the art for predictions over very extended periods of time, achieving 45% more accurate prediction performance at 50s compared to the baseline.
@INPROCEEDINGS{10342031, author={Zhu, Yufei and Rudenko, Andrey and Kucner, Tomasz P. and Palmieri, Luigi and Arras, Kai O. and Lilienthal, Achim J. and Magnusson, Martin}, booktitle={2023 IEEE/RSJ International Conference on Intelligent Robots and Systems (IROS)}, title={CLiFF-LHMP: Using Spatial Dynamics Patterns for Long- Term Human Motion Prediction}, year={2023}, volume={}, number={}, pages={3795-3802}, keywords={Tracking;Service robots;Dynamics;Green products;Prediction methods;Stairs;Probabilistic logic}, doi={10.1109/IROS55552.2023.10342031}}