Sariah Mghames, Luca Castri, Marc Hanheide, and Nicola Bellotto
Proceedings of the International Joint Conference on Neural Networks (IJCNN)
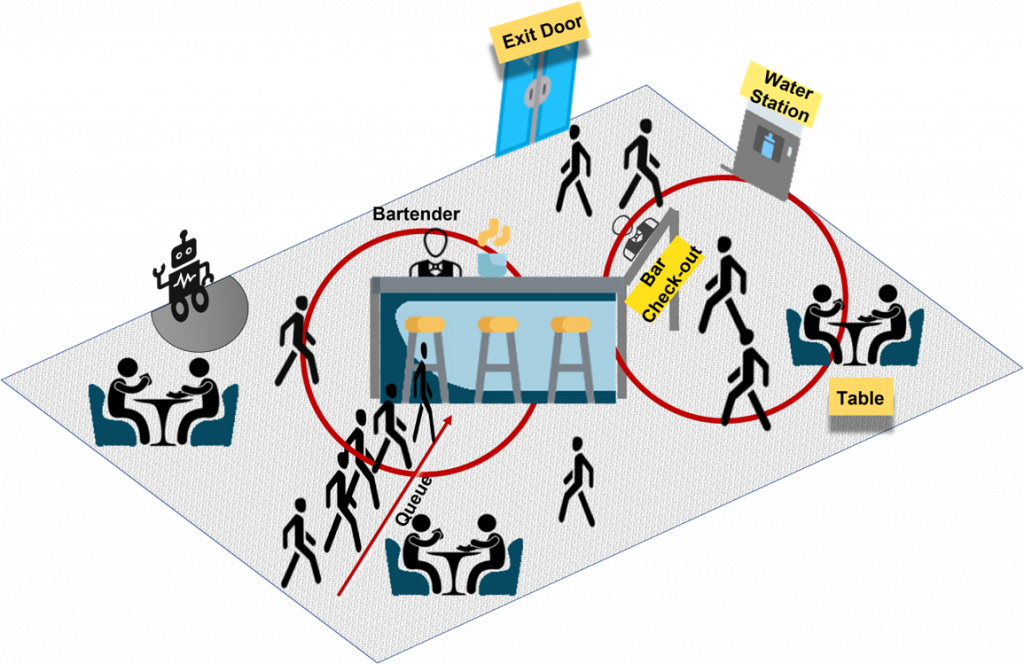
Abstract
Reasoning on the context of human beings is crucial for many real-world applications especially for those deploying autonomous systems (e.g. robots). In this paper, we present a new approach for context reasoning to further advance the field of human motion prediction. We therefore propose a neuro-symbolic approach for human motion prediction (NeuroSyM), which weights differently the interactions in the neighbourhood by leveraging an intuitive technique for spatial representation called Qualitative Trajectory Calculus (QTC). The proposed approach is experimentally tested on medium and long term time horizons using two architectures from the state of art, one of which is a baseline for human motion prediction and the other is a baseline for generic multivariate time-series prediction. Six datasets of challenging crowded scenarios, collected from both fixed and mobile cameras, were used for testing. Experimental results show that the NeuroSyM approach outperforms in most cases the baseline architectures in terms of prediction accuracy.
@inproceedings{sariah2023neuro, title={A Neuro-Symbolic Approach for Enhanced Human Motion Prediction}, author={Sariah, Mghames and Luca, Castri and Marc, Hanheide and Bellotto, Nicola and others}, booktitle={Proceedings of the International Joint Conference on Neural Networks (IJCNN)}, year={2023} }