Rishabh Jain, Narunas Vaskevicius, and Thomas Brox
Workshop onTransformers for Vision at IEEE Conference on Computer Vision and Pattern Recognition (CVPR)
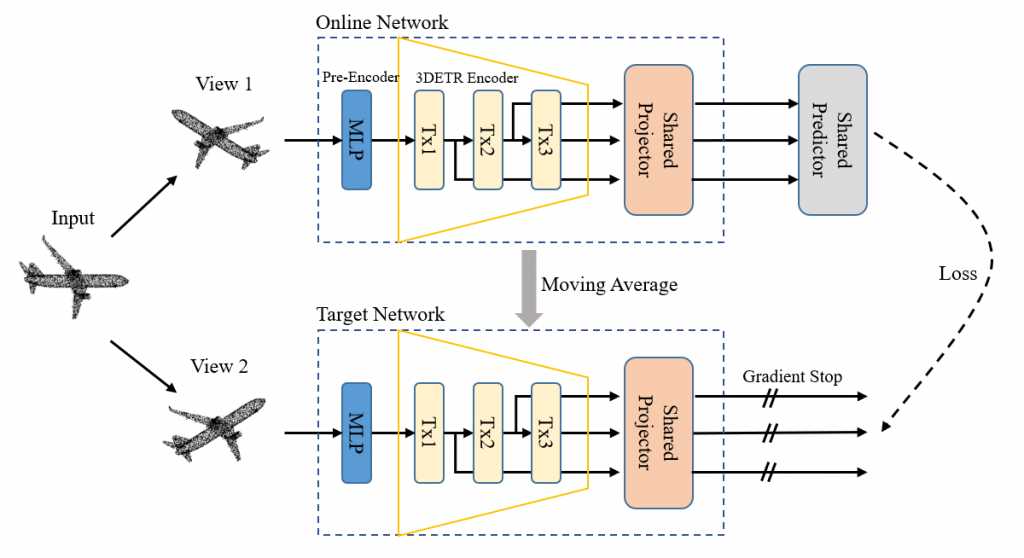
Abstract
3D Detection Transformer (3DETR) is a recent end-to-end transformer architecture for 3D object detection in 3D point clouds. In this work, we explore training and evaluation of 3DETR in a label-efficient setting on the popular 3D object detection benchmark SUN RGB-D. The performance of 3DETR declines drastically with decreasing amount of labeled data. Therefore, we investigate self-supervised pre-training of the 3DETR encoder with the spatio-temporal representation learning (STRL) framework. Opposite to our expectations, we observe that straightforward application of this framework leads to degraded representations which in some cases can even impair learning of the downstream task. To remedy this issue we extend STRL framework by introducing an auxiliary loss, which is applied to intermediate transformer layers. Our experiments demonstrate that this extension enables successful pre-training of 3DETR encoder and significantly boosts its label efficiency in the 3D object detection task.
@InProceedings{JainCVPR2022workshop, author = {Jain, Rishabh and Vaskevicius, Narunas and Brox, Thomas}, title = {Towards Self-Supervised Pre-Training of 3DETR for Label-Efficient 3D Object Detection}, booktitle = {Workshop on Transformers for Vision at IEEE Conference on Computer Vision and Pattern Recognition (CVPR)}, year = {2022}, }