Andrey Rudenko, Luigi Palmieri, Wanting Huang, Achim J Lilienthal, and Kai O. Arras
Proc. of the IEEE Int. Symp. on Robot and Human Interactive Comm. (RO-MAN)
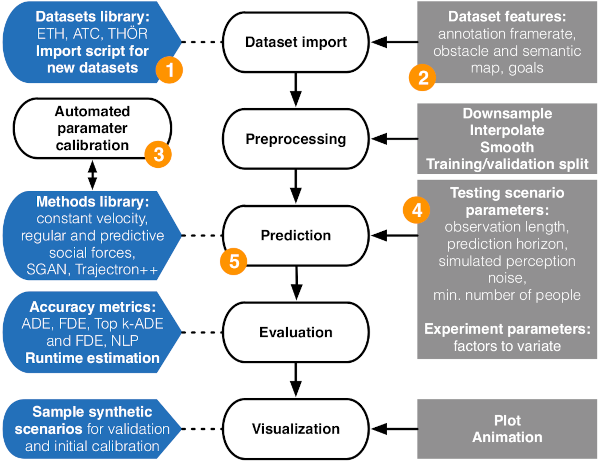
Abstract
Human motion trajectory prediction, an essential task for autonomous systems in many domains, has been on the rise in recent years. With a multitude of new methods proposed by different communities, the lack of standardized benchmarks and objective comparisons is increasingly becoming a major limitation to assess progress and guide further research. Existing benchmarks are limited in their scope and flexibility to conduct relevant experiments and to account for contextual cues of agents and environments. In this paper we present Atlas, a
benchmark to systematically evaluate human motion trajectory prediction algorithms in a unified framework. Atlas offers data preprocessing functions, hyperparameter optimization, comes with popular datasets and has the flexibility to setup and conduct underexplored yet relevant experiments to analyze a method’s accuracy and robustness. In an example application of Atlas, we compare five popular model- and learning-based predictors and find that, when properly applied, early physicsbased approaches are still remarkably competitive. Such results confirm the necessity of benchmarks like Atlas.
@inproceedings{rudenko2022atlas, title={The Atlas Benchmark: an Automated Evaluation Framework for Human Motion Prediction}, author={Rudenko, Andrey and Palmieri, Luigi and Huang, Wanting and Lilienthal, Achim J and Arras, Kai O}, booktitle={"Proc.~of the {IEEE} Int. Symp. on Robot and Human Interactive Comm. (RO-MAN)"}, year={2022}, }