Yash Goel, Narunas Vaskevicius, Luigi Palmieri, Chebrolu Nived, and Stachniss Cyrill
Workshop on Perception and Navigation for Autonomous Robotics in Unstructured and
Dynamic Environments at IEEE International Conference on Intelligent Robot sand Systems (IROS) (to appear)
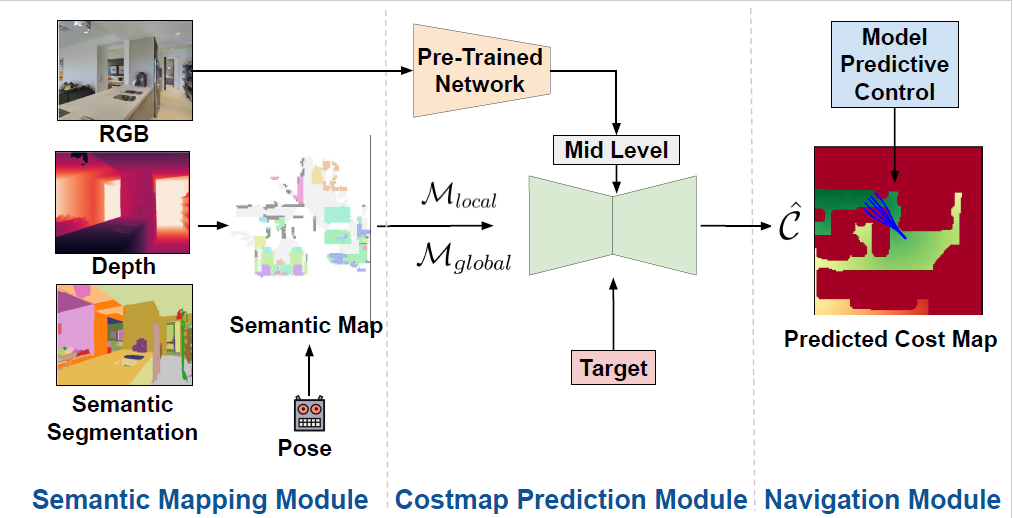
Abstract
We investigate the task of object goal navigation in unknown environments where the target is specified by a semantic label (e.g. find a couch). Such a navigation task is especially challenging as it requires understanding of semantic context in diverse settings. Most of the prior work tackles this problem under the assumption of a discrete action policy whereas we present an approach with continuous control which brings it closer to real world applications. We propose a deep neural network architecture and loss function to predict dense cost maps that implicitly contain semantic context and guide the robot towards the semantic goal. We also present a novel way of fusing mid-level visual representations in our architecture to provide additional semantic cues for cost map prediction. The estimated cost maps are then used by a sampling-based model predictive controller (MPC) for generating continuous robot actions. The preliminary experiments suggest that the cost maps generated by our network are suitable for the MPC and can guide the agent to the semantic goal more efficiently than a baseline approach. The results also indicate the importance of mid-level representations for navigation by improving the success rate by 7 percentage points.
@inproceedings{goel2022predicting, author = {Yash Goel and Narunas Vaskevicius and Luigi Palmieri and Nived Chebrolu and Cyrill Stachniss}, title = {Predicting Dense and Context-aware Cost Maps for Semantic Robot Navigation}, booktitle = {IROS 2022 Workshop on Perception and Navigation for Autonomous Robotics in Unstructured and Dynamic Environments} , year = {2022}, keywords={wp2} }