Marco Baracca, Giuseppe Averta, and Matteo Bianchi
Proceedings of the I-RIM Conference 2022
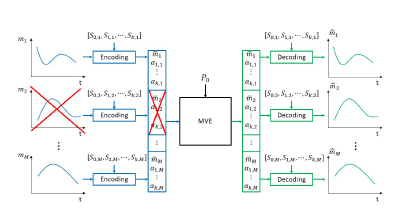
Abstract
Recording the level of muscles activation while human perform daily-living activities is a key factor to obtain a comprehensive evaluation of the biomechanical state. This type of analysis is especially important in work environments to identify and then avoid possible risky behaviours which could lead to work-related musculoskeletal disorders. Usually, muscular activation is recorded via wearable electromyographic EMG sensors. However, to achieve a whole-body state estimation a large number of sensing elements is necessary. This leads to uncomfortable and very expensive setups that prevent their adoption for monitoring daily working activities. To overcome this problem, we propose a solution to provide a reliable estimation of muscular activation from a limited number of EMG recordings. Our method exploits the covariation patterns between muscular activation signals to complement the recordings coming from a reduced set of optimally placed sensors, minimizing the estimation uncertainty. We tested this approach with a dataset containing EMG data from 10 different subjects. We were able to reconstruct the temporal evolution of 10 whole-body muscular activations with only 7 sensor elements achieving a maximum normalized estimation error of 13%.
@inproceedings{averta2022optimal, title=Optimal electromyographic sensing for whole-body muscular activity estimation, author=Marco Baracca, Giuseppe Averta and Matteo Bianchi, booktitle=2022 I-RIM Conference, DOI=10.5281/zenodo.7531398, pages={239--242}, year = 2022, organization=I-RIM }