Lorenzo Collodi, Davide Bacciu, Matteo Bianchi, and Giuseppe Averta
Learning with few examples the semantic description of novel human-inspired grasp strategies from RGB data
IEEE Robotics and Automation Letters
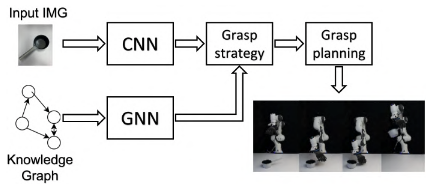
Abstract
Data-driven approaches and human inspiration are fundamental to endow robotic manipulators with advanced autonomous grasping capabilities. However, to capitalize upon these two pillars, several aspects need to be considered, which include the number of human examples used for training; the need for having in advance all the required information for classification (hardly feasible in unstructured environments); the trade-off between the task performance and the processing cost. In this paper, we propose a RGB-based pipeline that can identify the object to be grasped and guide the actual execution of the grasping primitive selected through a combination of Convolutional and Gated Graph Neural Networks. We consider a set of human-inspired grasp strategies, which are afforded by the geometrical properties of the objects and identified from a human grasping taxonomy, and propose to learn new grasping skills with only a few examples. We test our framework with a manipulator endowed with an under-actuated soft robotic hand. Even though we use only 2D information to reduce the footprint of the network, we achieve 90% of successful identifications of the most appropriate human-inspired grasping strategy over ten different classes, of which three were few-shot learned, outperforming an ideal model trained with all the classes, in sample-scarce conditions.
@Article{collodi2022learning, author = {Collodi,Lorenzo and Bacciu,Davide and Bianchi,Matteo and Averta,Giuseppe}, date = {2022-04}, journal = {IEEE Robotics and Automation Letters}, volume = 7, number = 2, title = {Learning with few examples the semantic description of novel human-inspired grasp strategies from {RGB} data}, }