Manuel Castellano-Quero, Tomasz Piotr Kucner, and Martin Magnusson
13th IROS Workshop on Planning, Perception, Navigation for Intelligent Vehicles
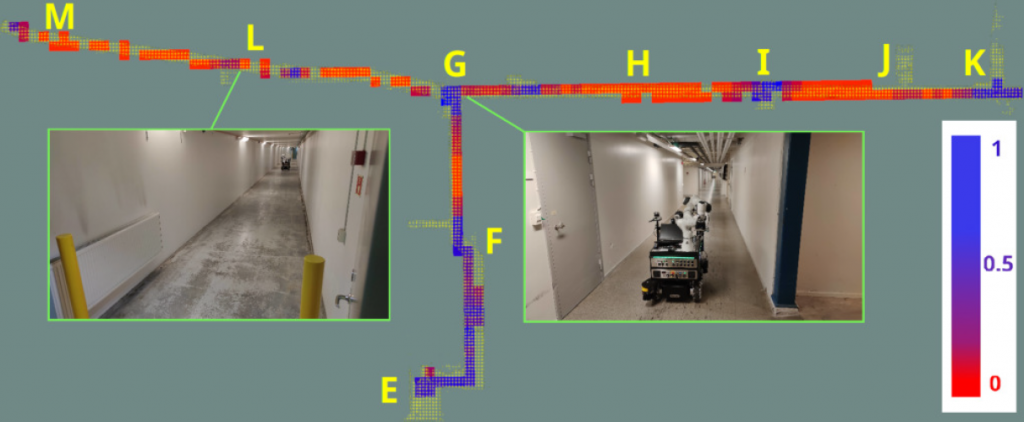
Abstract
Ensuring precise localization is key to most mobile-robot systems, not least those that are deployed in industrial settings. However, even state-of-the art lidar-based systems may fail or lose accuracy, in particular in feature-sparse environments (e.g. transport corridors and fully stacked warehouse aisles). In this paper, we introduce alignability maps that spatially capture localization risk and predict where in a map localization may be less accurate. Our proposal is based on the concept of alignability, which represents the capacity of a given range scan to be aligned with subsequent ones. We explore different measures of alignability and evaluate them in terms of their ability to predict localization error. Our experiments show that alignability maps, which we deploy in different industrially relevant environments, serve to predict the quality of localization. Finally, we also integrate our approach in a motion planning use case. Our results demonstrate that a planner can incorporate our alignability maps as cost maps to generate paths with lower risk of localization error.
@inproceedings{castellano2023alignability, title={Alignability maps for the prediction and mitigation of localization error}, author={Castellano-Quero, Manuel and Kucner, Tomasz Piotr and Magnusson, Martin}, booktitle={IROS 2023 Workshop: Closing the Loop on Localization}, year={2023} }