Nico Mansfeld, Manuel Keppler and Sami Haddadin
IEEE Robotics and Automation Letters
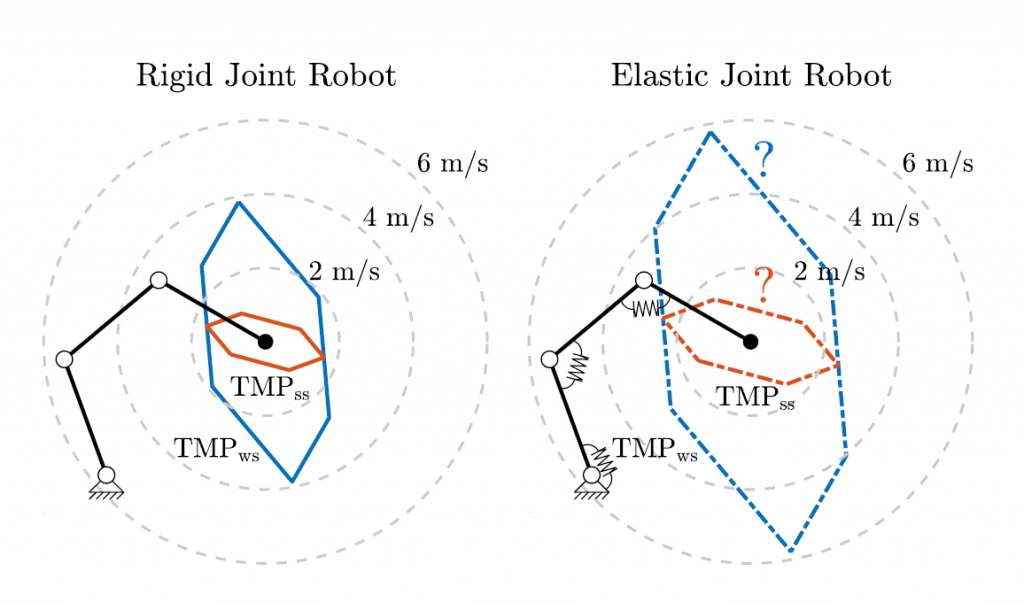
Abstract
Estimating the 6DOF pose of objects is an important function in many applications, such as robot manipulation or augmented reality. However, accurate and fast pose estimation from 3D point clouds is challenging, because of the complexity of object shapes, measurement noise, and presence of occlusions. We address this challenging task using an end-to-end learning approach for object pose estimation given a raw point cloud input. Our architecture pools geometric features together using a self-attention mechanism and adopts a deep Hough voting scheme for pose proposal generation. To build robustness to occlusion, the proposed network generates candidates by casting votes and accumulating evidence for object locations. Specifi- cally, our model learns higher-level features by leveraging the dependency of object parts and object instances, thereby boosting the performance of object pose estimation. Our experiments show that our method outperforms state-of-the-art approaches in public benchmarks including the Siléane dataset and the Fraunhofer IPA dataset. We also deploy our proposed method to a real robot pick-and-place based on the estimated pose.
@ARTICLE{mansfeld2021speed, author={Mansfeld, Nico and Keppler, Manuel and Haddadin, Sami}, journal={IEEE Robotics and Automation Letters}, title={Speed Gain in Elastic Joint Robots: An Energy Conversion-Based Approach}, year={2021}, volume={6}, number={3}, pages={4600-4607}, doi={10.1109/LRA.2021.3068698} }