Edmundo Fortunić, Abdalla Swikir, Saeed Abdolsah, and Sami Haddadin
Proceedings of 2022 IEEE/RSJ International Conference
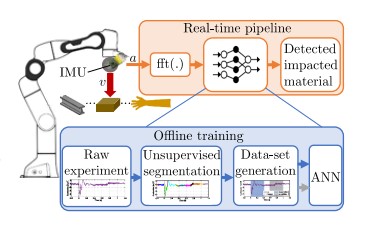
Abstract
In modern highly dynamic robot manipulation, collisions between a robot and objects may be intentionally executed to improve performance. To distinguish between these deliberate contacts and accidental collisions beyond the limit of state-of-the-art human-robot interactions, new sensing approaches are required. This work seeks an easy-to implement and real-time capable solution to detect the identity of the impacted material. We developed an inertial measurement unit (IMU) based setup that records vibration signals occurring after collisions. Furthermore, a data-set was generated in an unsupervised learning manner using the measurements of collision experiments with several materials commonly used in realistic applications. The data-set was used to train an artificial neural network to classify the type of material involved. Our results show that the neural net detects collisions and a detailed distinction between materials is achieved, even with estimating different human body parts. The unsupervised dataset generation allows for a simple integration of new classes, which provides broader applicability of our approach. As the calculations are running faster than the control cycle of the robot, the output of our classifier can be used in real-time to decide about the robots reaction behavior.
@inproceedings{inproceedings, author = {Fortunić, Edmundo and Swikir, Abdalla and Abdolsah, Saeed and Haddadin, Sami}, year = {2022}, month = {06}, pages = {}, title = {Real-time IMU-Based Learning: a Classification of Contact Materials} }